In today's digital age, we are inundated with vast amounts of data generated from various sources, including social media, sensors, and transaction records. Amidst this data deluge lies a wealth of untapped knowledge waiting to be discovered. This is where data mining comes into play.
What is Data Mining?
Data mining is the process of extracting valuable insights, patterns, and knowledge from large datasets using a combination of statistical methods, machine learning techniques, and computational algorithms. It involves uncovering hidden patterns, trends, and relationships within the data to facilitate decision-making and drive innovation.
Key Concepts in Data Mining:
Data Preprocessing:
Before diving into analysis, raw data often requires preprocessing to clean, transform, and integrate disparate datasets. This ensures that the data is suitable for analysis and free from inconsistencies or errors that could distort the results.
Exploratory Data Analysis (EDA):
Exploratory data analysis involves visualizing and Chinese Overseas Europe Number summarizing data to gain insights into its characteristics and underlying structure. Techniques such as histograms, scatter plots, and summary statistics help analysts understand the distribution, relationships, and outliers within the data.
Model Building and Evaluation:
Data mining encompasses building predictive models or uncovering patterns using algorithms such as decision trees, neural networks, clustering, and association rule mining. These models are evaluated using various metrics to assess their performance and generalization capabilities.
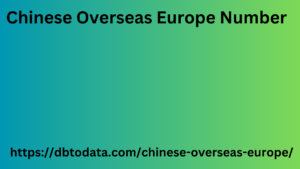
Pattern Recognition and Prediction:
One of the primary goals of data mining is to recognize patterns and make predictions based on historical data. Whether it's predicting customer behavior, identifying fraudulent activities, or forecasting future trends, data mining enables organizations to leverage data-driven insights for strategic decision-making.
Applications of Data Mining:
Data mining finds applications across diverse domains, including business, healthcare, finance, marketing, and cybersecurity. It aids in customer segmentation, market basket analysis, personalized medicine, risk assessment, and anomaly detection, among other use cases.
Challenges and Considerations:
Despite its immense potential, data mining poses several challenges, including data quality issues, computational complexity, ethical considerations, and privacy concerns. Organizations must address these challenges responsibly to ensure the ethical and effective use of data mining techniques.
Conclusion:
In conclusion, data mining serves as a powerful tool for transforming raw data into actionable insights, driving innovation, and enhancing decision-making across various industries. By leveraging statistical methods, machine learning algorithms, and computational techniques, organizations can unlock the hidden knowledge buried within their datasets, paving the way for a data-driven future.